Uh oh, 404!
Marcus here looks after our website, so it's his responsibility the page you were looking for can't be found. He'll be banned from the table tennis table in the office for this, unless you want to take pity on him?
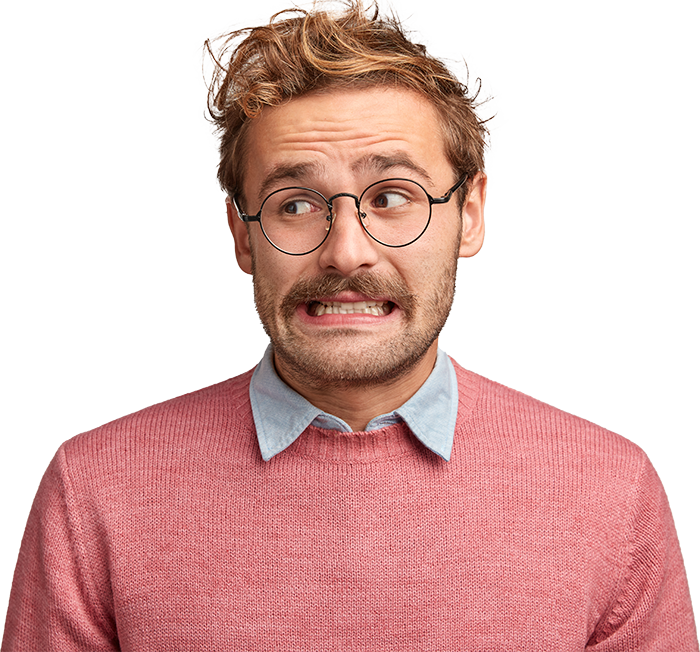
Marcus here looks after our website, so it's his responsibility the page you were looking for can't be found. He'll be banned from the table tennis table in the office for this, unless you want to take pity on him?